Our Research
Research
MMLab@NTU
Members in MMLab@NTU conduct research primarily in low-level vision, image and video understanding, creative content creation, 3D scene understanding and reconstruction.
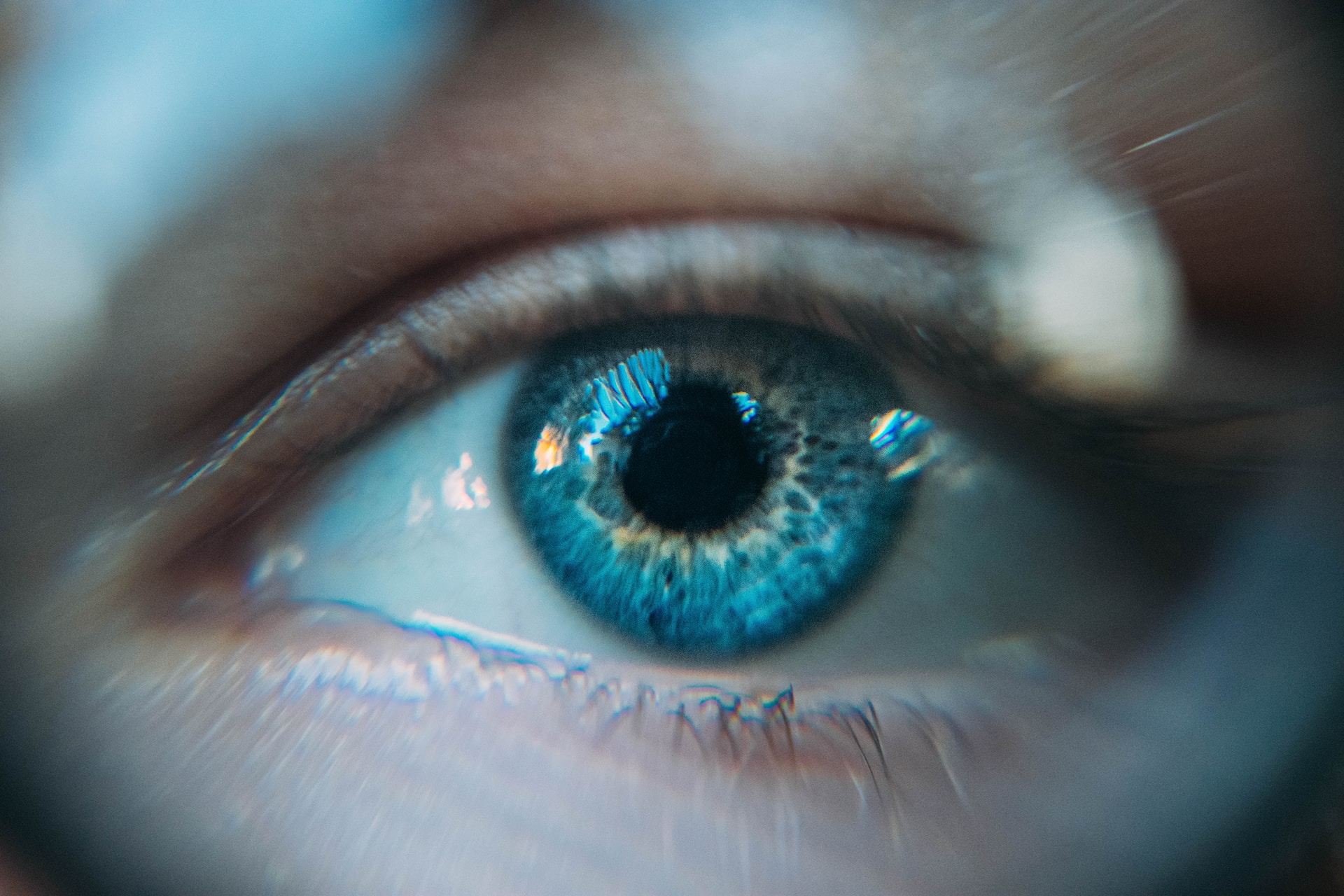
Super-Resolution
Our team is the first to introduce the use of deep neural networks to directly predict super-resolved images. Our journal paper on image super-resolution was selected as the `Most Popular Article' by IEEE Transactions on Pattern Analysis and Machine Intelligence in 2016. It remains as one of the top articles to date. Popular image and video super-resolution methods developed by our team include SRCNN, ESRGAN, EDVR, GLEAN, BasicVSR and CodeFormer.
Content Editing and Creation
We research new methods for generating high-resolution, realistic and novel contents in images and videos. We are also interested in investigating fundamental concepts in generative models. Some of our works include diffusion models, face generation and editing, high-resolution portrait video style transfer, video synthesis, human image generation, and style transfer.
Image and Video Understanding
We explore effective and efficient methods to detect, segment and recognize objects in complex scenes. We were the champion in COCO 2019 Object Detection Challenge 2019, and Open Images Challenge 2019. Some of our recent projects focus on open-vocabulary detection and segmentation, learning universal visual representation, multitasks models, and building multimodality models such as Otter.
3D Generative AI
Our team has been working on various tasks related to 3D generative AI to enable the creation of high-quality 3D models. Check out our recent work on novel view syntensis with free camera trajectories, OmniObject3D - a large vocabulary 3D object dataset with massive high-quality real-scanned 3D objects, algorithms to generate immersive 3D scenes, 3D avatar generation, and high-quality shape and texture reconstruction.
Deep Learning
We investigate new deep learning methods that are more efficient, robust, accurate, scalable, transferable, and explainable. We have been working on various problems like domain generalization, knowledge distillation, long-tailed recognition, and self-supervised learning. We participated in Facebook AI Self-Supervision Challenge 2019, and we won champions of all four tracks. Check out MMSelfSup, our open-source project for self-supervised learning.
Media Forensics
The popularization of Deepfakes on the internet has set off alarm bells among the general public and authorities, in view of the conceivable perilous implications. We have proposed two large-scale datasets for face forgery detection. See DeeperForensics and ForgeryNet. Also check out our recent work on detecting multimodal media manipulation.
Research
Video Demos
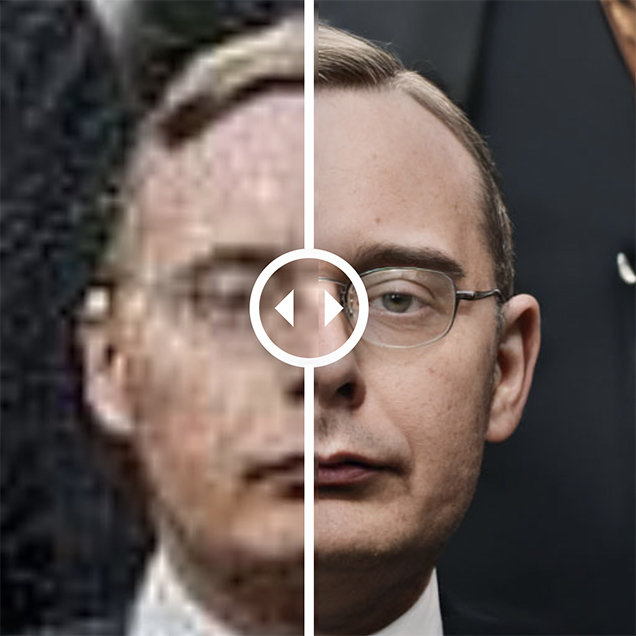
CodeFormer (Hugging Face Demo)
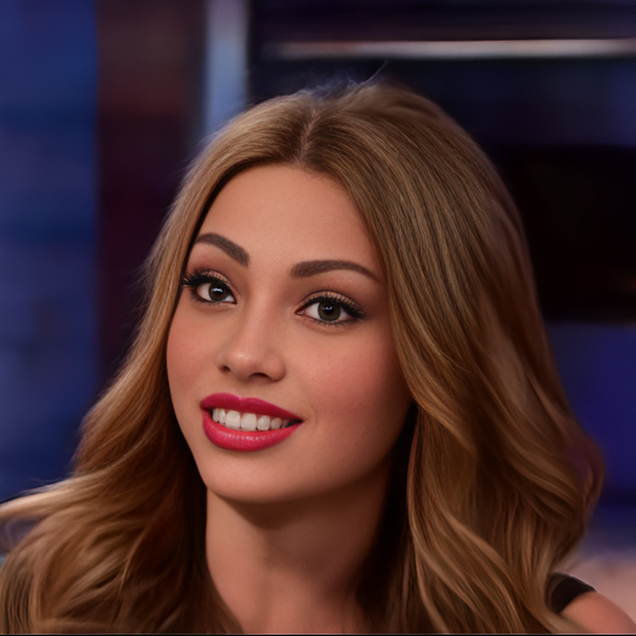
VToonify (Hugging Face Demo)
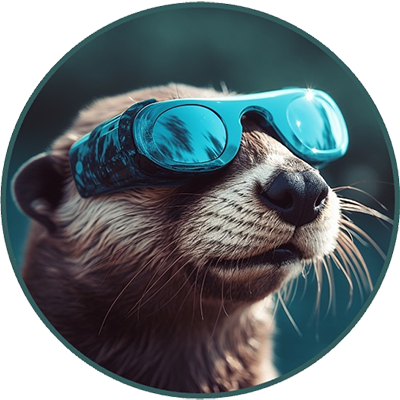
Otter Chatbot (Demo)
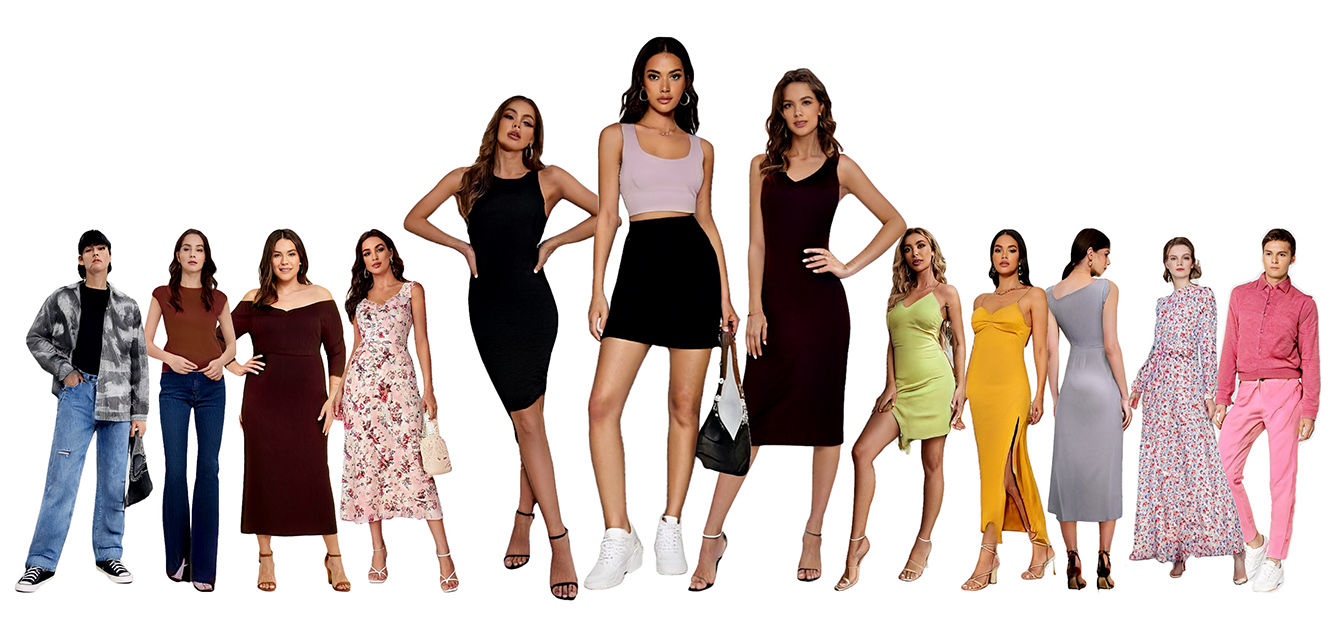
Human Image Generation (Hugging Face Demo)
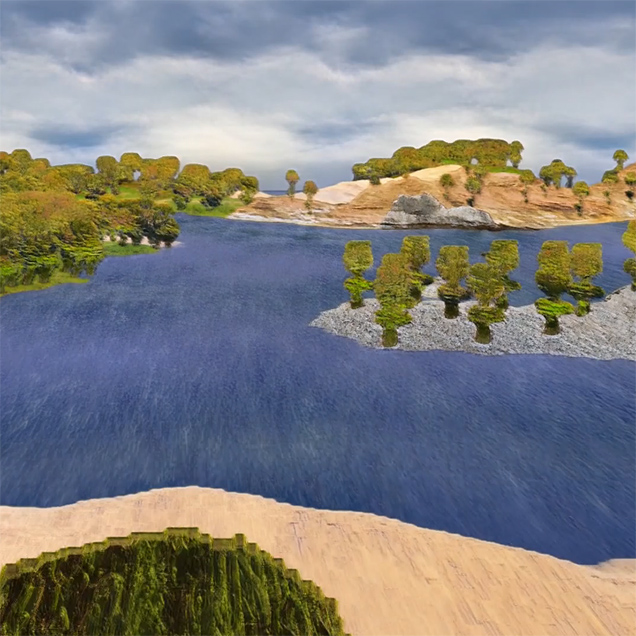
SceneDreamer (Hugging Face Demo)
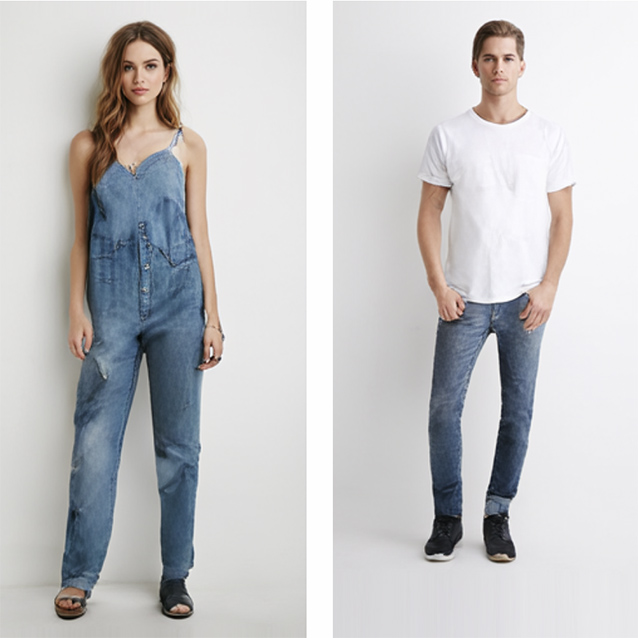
Text2Human (Hugging Face Demo)
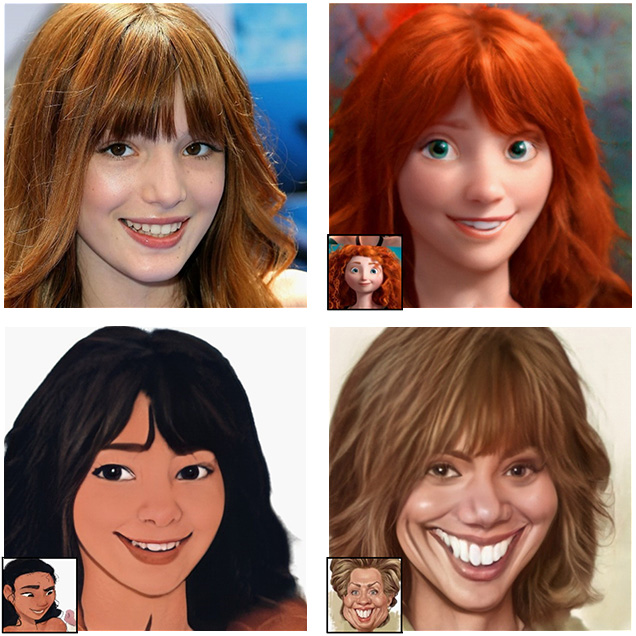
Portrait Style Transfer (Hugging Face Demo)
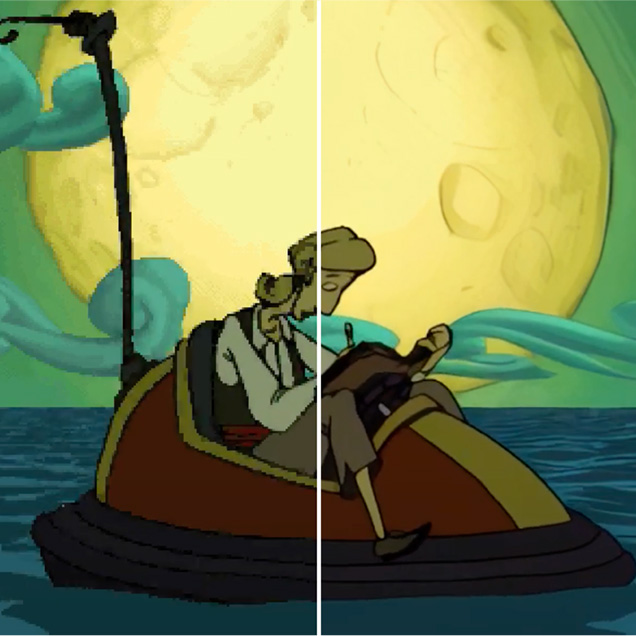
Anime Super-Resolution (Credit: Jan Bing)
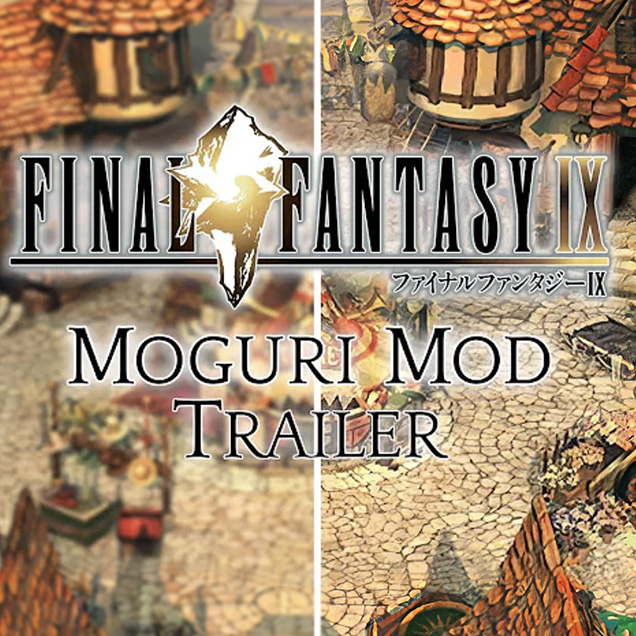
Game Super-Resolution (Credit: Snouz)
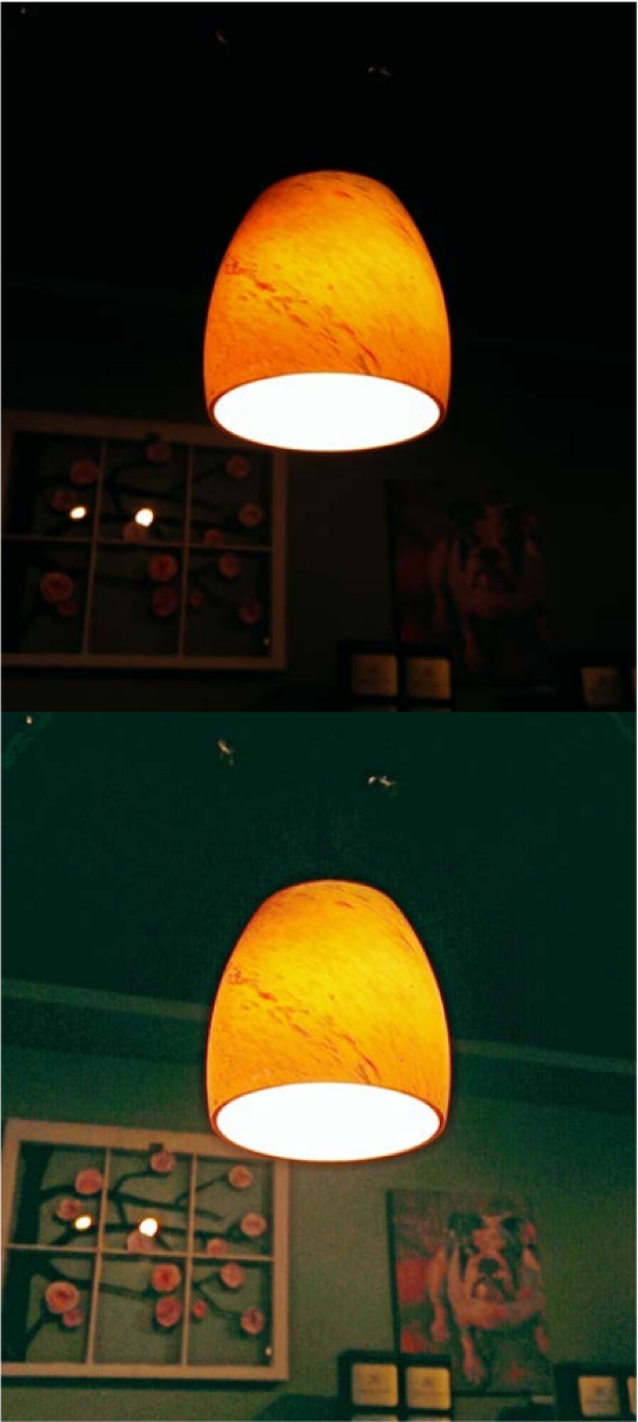
Low-Light Enhancement (Hugging Face Demo)
Selected
Media Coverage | Blog
- Artificial Intelligence Is Helping Old Video Games Look Like New
By James Vincent in The Verge - Meet the Upscalers
By Daniel Cooper in Engadget - AI Neural Networks Are Giving Final Fantasy 7 a Makeover
By Fraser Brown in PC Gamer - AI Is About to Transform The Future (And Past) of Video Games
By Aidan Moher in Input Mag - Final Fantasy IX Recreated
By Moguri Mod
Source: @Luke_Aaron